Our Mission
UChicago STEM Education connects research and practice to improve education for all.
37
years in existence
2.1M
# of people using
our tools daily
our tools daily
31
current projects
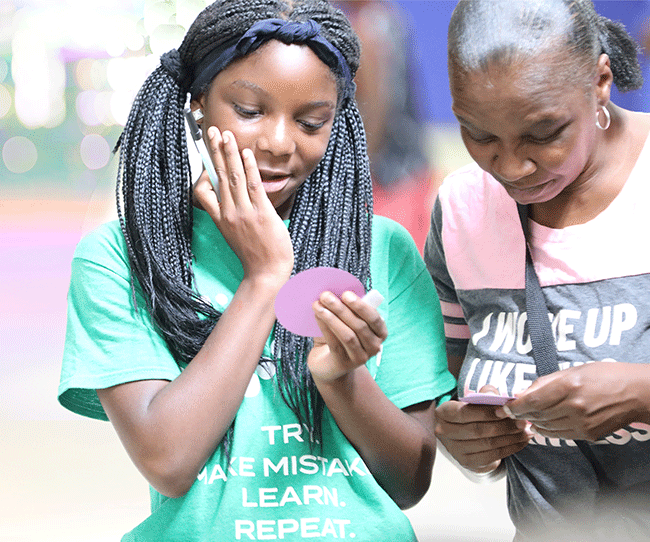
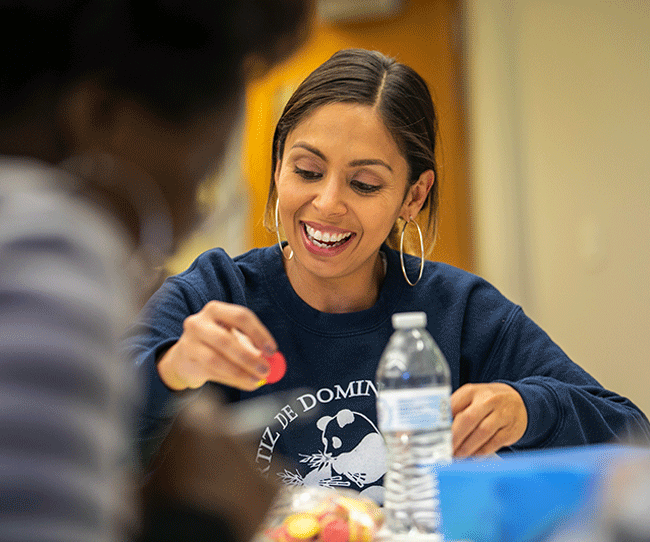
School Support Services
Supporting Schools to Make Lasting Change
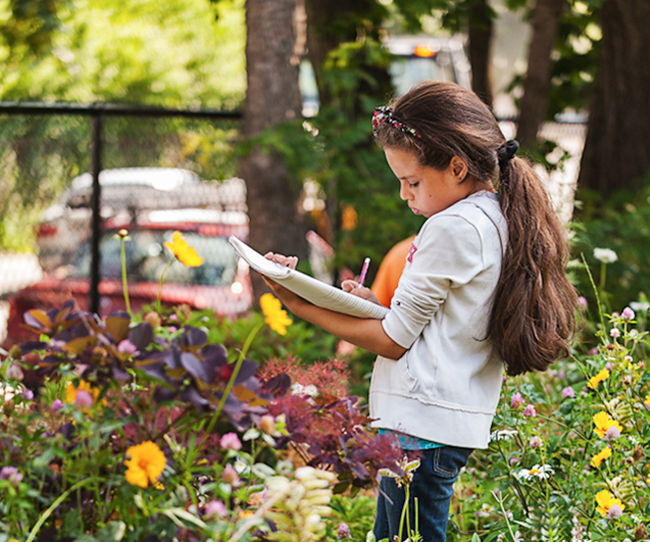
Research & Evaluation
Generating Knowledge for Improvement and Impact
Funders
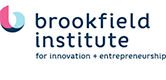
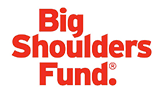
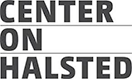
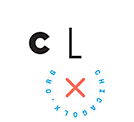
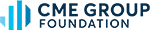

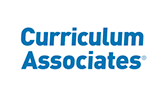

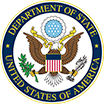

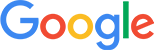
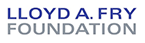
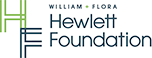
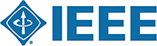

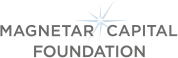

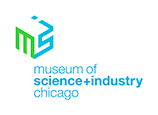

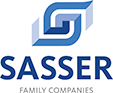
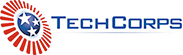
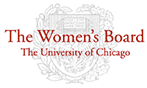
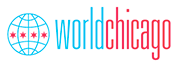
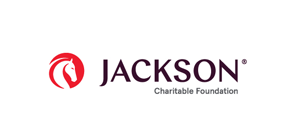